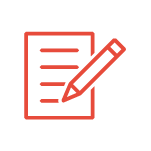
Summary
The explosive growth of socially-sensed geolocated data (i.e., geosocial data) has offered us an unprecedented opportunity to model global land use dynamics at varying spatial scales, semantic granularities and temporal resolutions. Geosocial data (e.g., geotagged social media, user-generated ground images, POI data), with its universal availability and important role in daily human activities, has been widely used for land use characterization. MapSpace aims develop a time-variant global land use model with multiple spatial scales and semantic granularities. The first phase of MapSpace has developed a POI-based global land use modeling framework based on global POI data from the PlanetSense project. The combination of spatial distribution, semantic characteristics, and sometimes temporal dynamics of POIs inside an AOI can capture its unique land use characteristics. Heterogeneous POIs data sources were fused using a unified semantic representation framework and combined with a neural network language model to generate spatially explicit AOI embedding at different spatial scales. Road network hierarchy was incorporated into the neural network language model to integrate spatial dimension and semantic dimension of POIs. The scalable land use modeling framework can be easily extended to incorporate other geosocial data sources and efficiently produce global land use map at different spatial scales and semantic granularities.
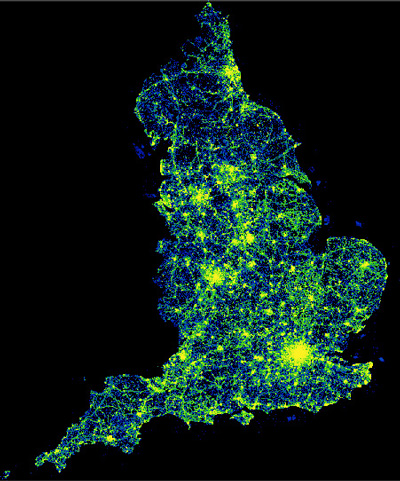
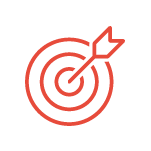
Objectives
- Link human activities with place characteristics
- Characterizing semantic motif of places based on geosocial data
- Global land use classification at different spatial scales and semantic granularities
- Capture temporal dynamics of land use
- Extensible land use modeling framework that can incorporate diverse social, economic and demographic data
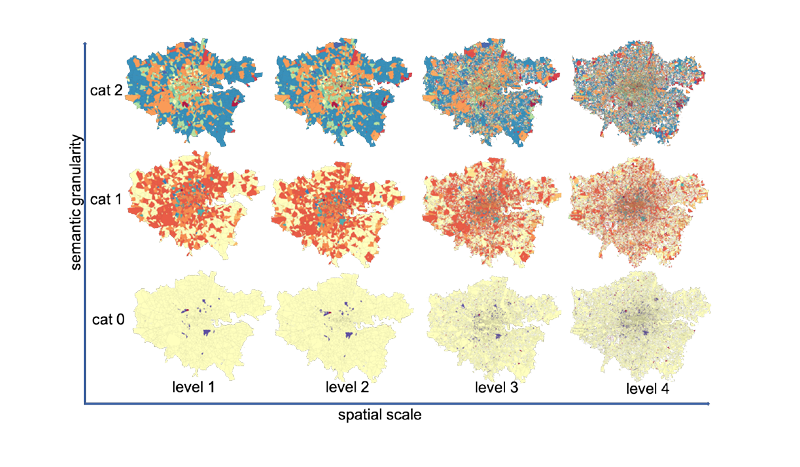
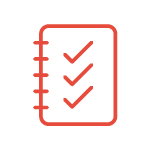
Features
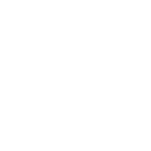
Heterogeneous POIs data sources were fused based on a unified semantic representation framework.
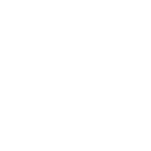
Spatially explicit AOI embedding that integrate spatial and semantic dimensions of POIs
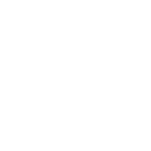
Data-driven approach to identify the most appropriate spatial scale and semantic granularity for different geographic region
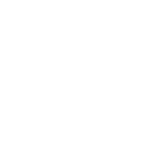
An easy-to-extend land use modeling framework that can further incorporate temporal and real-time dynamics of places
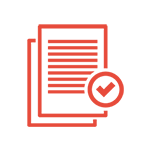
Publications
Year | Citation |
---|---|
2023 | Fan, Junchuan & Thakur, Gautam (2023), POI-based land use map for Africa, Dryad, Dataset, https://doi.org/10.5061/dryad.hhmgqnkk6 |
2023 | Fan, J., & Thakur, G. (2023). Towards POI-based large-scale land use modeling: spatial scale, semantic granularity and geographic context. International Journal of Digital Earth, 16(1), 430–445. https://doi.org/10.1080/17538947.2023.2174607 |
2021 | Thakur, G., & Fan, J. (2021). MapSpace: POI-based Multi-Scale Global Land Use Modeling. GIScience Conference 2021. https://doi.org/10.25436/E2Z59N |
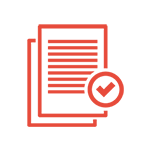
News
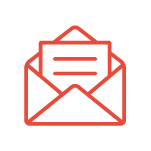
Contact Us
Junchuan Fan
Location Intelligence Group
Human Dynamics Section,
Geospatial Science and Human Security Division National Security and Sciences Directorate
Oak Ridge National Laboratory
Gautam Thakur
Location Intelligence Group
Human Dynamics Section,
Geospatial Science and Human Security Division National Security and Sciences Directorate
Oak Ridge National Laboratory